Predictive maintenance with IoT: The road to real returns
The Internet of Things is having a profound effect on the manufacturing sector, leading to increased automation, more efficient operations, and the creation of valuable new business models. While the application of digital technologies can bring benefits across the value-chain, it is arguably in the area of predictive maintenance that the most significant impact can be derived.
The use of sensors and data analysis means companies can spot patterns in equipment condition and performance, and accurately predict when a failure might occur. Such foresight eliminates unplanned downtime, delivering substantial productivity benefits.
The business case for predictive maintenance with IoT
Before we outline how IoT can underpin the implementation of predictive maintenance, it’s useful to set some context by looking at how maintenance activities have traditionally been performed. In most cases, schedule-based maintenance using predetermined intervals has been the most common method of reducing the likelihood of equipment and plant failure. There’s a problem with that, though - age isn’t always an accurate indicator of condition. So, this preventative approach has often resulted in parts or machinery being replaced after a specific period even though they could have lasted much longer.
Indeed, this type of maintenance assumes a higher likelihood of failure with increased use or age. However, according to research from the ARC Advisory Group, this only applies to 18 percent of industrial assets, with the other 82 percent displaying a random failure pattern. This inevitably results in unplanned downtime and lost productivity, along with other less obvious factors which need to be considered too.
Random failure can cause damage to other associated equipment, and with that comes safety risks. Also, unplanned downtime requires urgent rectification, and that can result in higher salary payments through unexpected overtime and other associated costs. In short, preventative maintenance has some severe limitations that impact the bottom line.
So, what is needed is a genuinely predictive approach – using sensors, data collation, analytics and machine learning to monitor equipment continuously and predict failure with far greater accuracy. This kind of plan – utilising the full benefit of the Internet of Things - delivers maintenance that is necessary rather than possibly required. Moreover, in the future, some types of machines will be able to perform self-maintenance, eliminating the need for human intervention.
The size of the prize for predictive maintenance within manufacturing is immense.
According to management consulting firm McKinsey, predictive maintenance could reduce the costs of factory equipment by up to 40 per cent, while reducing downtime by up to 50 per cent. It also has the opportunity to reduce capital investment by up to 5 per cent, by extending the life of existing industrial assets. As a result, these savings could amount to a staggering $630 billion per year by 2025, predicts McKinsey. No wonder manufacturers are embracing IIoT-enabled predictive maintenance as a means of transforming their businesses.
The core technologies enabling predictive maintenance
So how does predictive maintenance work? At a top-line level, it’s delivered through the coming together of several mega-trends, most notably big data, cloud computing, edge computing, machine learning and connectivity. Then, the challenge for design engineers developing IoT solutions is to build a supporting platform using core products that are suitable for the specific task at hand. These products include sensors, wired and wireless solutions, antennas, batteries and increasingly smaller connectors and passive components to enable small, often remote, low-power connectivity. Also, these products will need to have been designed to withstand adverse conditions often found within industrial environments.
Once in place, sensor data can be taken from assets such as actuators, motors and drives, and filtered through field gateways, before being pushed on the cloud through wireless connectivity. The sensor data is then repacked, effectively, so it can be streamed in an orderly flow to a data lake for filtering. Once structured at a big data warehouse into more meaningful information relating to specific performance indicators, such as vibration or temperature, the data can be analysed with machine learning to identify any anomalies. As predictive models are built and trained over time, they become more accurate and therefore deliver more value.
What’s crucial here, of course, is to ensure that the right data is collected, and the right datasets are analysed. IoT data that’s used to determine the condition of a machine might cover factors such as temperature and vibration, while other static data feeding into the model might cover the specifics of make, model or configuration. Usage history data and service information can also be used to improve the effectiveness of the model and improve its predictive outcomes.
There also needs to be an element of contextual awareness, taking into account the complex static and dynamic variability of physical devices, often influenced by the specifics of the operating environment. By gradually recognising patterns and identifying abnormal behaviour in the context of the kinds of variable conditions found in industrial settings, machine learning software can more accurately understand long-term trends and spot undesired events before they cause downtime.
2 examples of IoT-enabled smarter maintenance
Such techniques are already being used to good effect in discrete manufacturing as part of initiatives to develop the smarter factories of the future. One example of this is Sandvik, the tooling and tooling systems manufacturer, which has teamed up with Microsoft to create sensorised cutting equipment. This combines data collection, streaming analytics and machine learning to notify engineers when tool bit maintenance is required, or to provide an alert of impending failure.
 |
Meanwhile, the engineering group ABB has developed a predictive maintenance solution for critical motor and drive applications in manufacturing environments. In this example, sensors, cloud computing and machine learning combine to provide an overview of equipment performance to keep production running as planned. This has been used to good effect at Tenaris, the steel pipe manufacturer in Italy, which has used the technology to monitor high and low-voltage motors running critical pumps and fans, 24/7. The predictive maintenance solution has been used to collect and analyse vibrations to indicate bearing failure, and voltage and power anomalies that indicate a short circuit.
The challenges of implementing predictive maintenance
If the concept of IIoT-enabled predictive maintenance is now well-understood, and some forward-thinking companies are using it within their plants, why is it that recent research shows that adoption across manufacturing has been slower than expected?
A survey of 600 high-tech executives by the global management consulting firm Bain and Company found that industrial customers were less excited about the potential of predictive maintenance in 2018 than they had been two years earlier. This shift in sentiment, said Bain and Company, had come about because manufacturers had found implementing predictive maintenance harder than they had expected, and that deriving insight from the data had proved more challenging than they had initially thought. As proof of concept projects had been set in motion, many of these companies had identified concerns over integration issues, particularly relating to a lack of technical expertise, data portability and transition risk.
The survey found that although manufacturers retained long-term enthusiasm for IIoT-enabled predictive maintenance, many companies were pausing for thought, as they recognised that implementation of digital projects might take longer than initially thought and that return on investment might be longer than expected.
Six tools for best-practice maintenance
This newfound sense of realism could well prove beneficial in the long-term. As the initial hype around predictive maintenance fades away, it’s likely to be replaced with more considered debate around the pros and cons of adoption. It also provides an opportunity to take stock and learn from best-practice advice from organisations that have led the way.
Hitachi, for example, has identified six primary tools and techniques that all successful predictive maintenance programs should have to make them function effectively and deliver a reasonable chance of success. They are:
- Small early pilot programs
- A technology suite for aggregating data
- Algorithms to monitor patterns and events in real time
- Effective workflows
- Service management
- A change management agreement
These best practices should assist engineers as they grapple with issues such as the business case and promised value of predictive maintenance, the technological and data requirements, and the challenges to full implementation and delivering on that promise.
Ultimately, IIoT-enabled predictive maintenance provides a brave new world for manufacturers looking to improve productivity, underpin safety and deliver lower costs. However, the journey to that end game will take longer, and have more ups and downs, than many people might have expected.
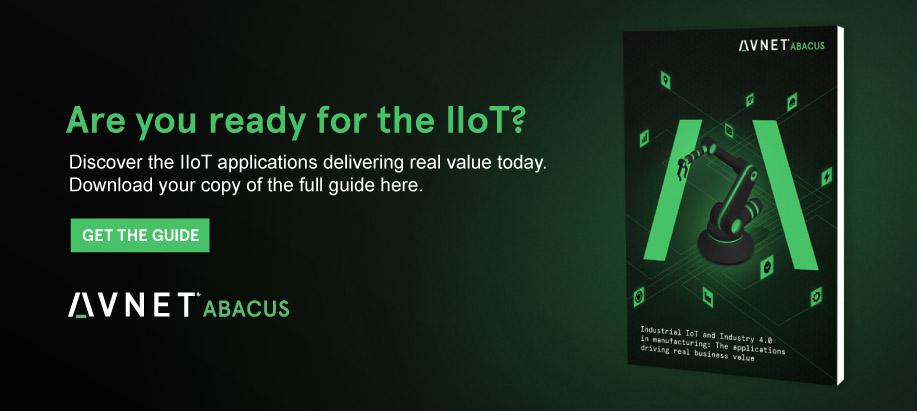