Greater Efficiency in Healthcare
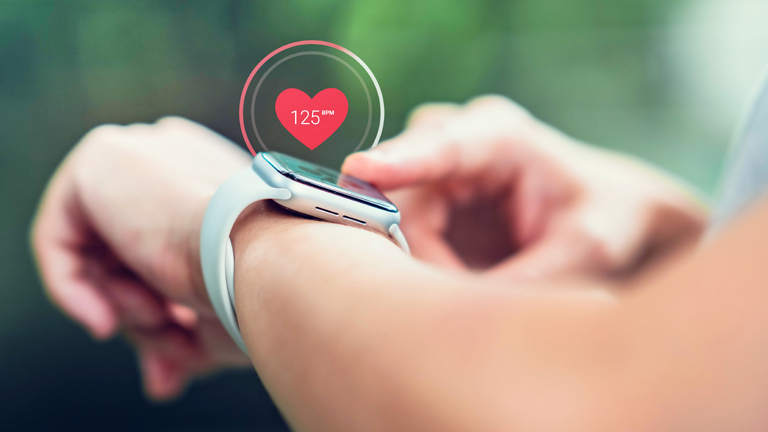
The healthcare sector is facing enormous challenges. Costs are exploding worldwide – market researchers at Deloitte expect that the global health spend in 2022 will rise to USD 10 trillion (in 2017, this figure was USD 7.7 trillion). Chronic diseases are arising with increasing frequency. According to the World Health Organization (WHO), thirteen million people worldwide die each year from cardiovascular diseases, chronic respiratory diseases, diabetes and cancer before reaching the age of 70. What’s more, it is becoming increasingly difficult to find the right personnel who can offer medical services on a comprehensive basis.
Wearables analyse vital parameters
One solution for overcoming these challenges is to use IoT edge devices and their underlying computer architectures. For example, wearable edge devices can collect, store and analyse critical patient data without having to be in constant contact with a network infrastructure. Such medical products therefore help diagnoses to be made quickly and easily without the patient necessarily having to attend a medical practice or a hospital. In addition, the information collected can be sent at regular intervals to the central servers in the cloud where it is checked by the attending physician or stored for long-term diagnoses.
Warning of asthma attacks
One example here is Adamm – a wearable intelligent asthma monitor that detects the symptoms of an asthma attack before it happens. The wearer can therefore take action before the situation deteriorates. The sensors in the wearable detect the patient’s individual symptoms – they monitor the cough rate, breathing pattern, heartbeat, temperature and other relevant data. The asthma monitor uses algorithms to learn the patient's “normal condition”. Its ability to detect when an attack is indicated therefore improves continually over time. All of the data is processed on the device itself. Whenever the data deviates from the patient’s individual norm, the wearable vibrates and thus informs the patient about the deviation. At the same time, Adamm can also send an SMS to a previously nominated nurse or person of trust. The device is not dependent on the computing power of a smartphone and therefore offers true autonomy. However, it can send data as needed to an app or a web portal.
Support for emergency call centers
AI-based edge devices not only offer assistance in terms of monitoring patient data: for example, Danish company Corti has developed a system that is able to support medical professionals, such as emergency dispatchers. Corti triage leverages an edge-computing device called Orb. Orb is part of Corti's real-time decision-making system, which uses AI technology to identify important patterns in the incoming emergency call and warns the dispatcher of events that require the swiftest possible response, such as a heart attack. The device is simply placed on the dispatcher’s table for this purpose. It connects to the audio stream of the telephone in order to monitor emergency calls. Instead of being explicitly pre-programmed with sample events, the AI algorithm learns to identify key factors by listening to large numbers of calls. The system also considers non-verbal sounds, which can supply important information. Edge computing not only offers the advantage of a very fast response, as Corti co-founder and CTO Lars Maaløe stresses: “Efficiency is crucial for edge devices – particularly in an emergency setting. And edge computing has the significant benefit of allowing the Orb to function continuously, even when the internet connection is interrupted.” By listening in on the phone, Corti's Orb should assist with reducing the number of undiscovered heart attacks by more than 50 per cent and detect within 50 seconds whether a heart attack has happened. This represents an important time gain because, from collapse to start of resuscitation, the chance of the caller surviving drops by ten per cent per minute: the dispatchers could therefore urgently do with some extra assistance in order to detect a cardiac arrest quickly and efficiently.
Enhanced MRI images
Edge technology also helps to improve services for patients in the hospital. For example, edge computing allows faster recording times, improved image quality and fewer discrepancies using magnetic resonance imaging machines from GE Healthcare. The MRI machines have embedded high-performance graphics processors for this purpose. Together with AIRx, an AIbased automated workflow tool for performing MRI brain scans, they enable automatic identification of anatomical structures. The system then independently determines the cutting point and angle of visual recordings for neurological examinations. This leads to fewer recording errors and significantly reduces the time the patient spends in the MRI machine. AIRx is based on Edison, a General Electric platform, which is intended to accelerate the development and roll-out of AI technology. Edison can integrate and assimilate data from different sources, apply advanced analyses and AI to transform data and generate appropriate findings on this basis. “AI is fundamental to achieving precision health and must be pervasively available from the cloud to the edge and directly on medical devices,” stresses Dr Jason Polzin, General Manager of MR Applications, GE Healthcare. “Real-time, critical-care use cases demand AI at the edge.”
****
This article is also available in German language. Click here for the German version.



